Theodore Brooks
I am Theodore Brooks, a computational biologist and AI systems architect dedicated to unraveling the complexity of human health through multimodal biomarker integration and dynamic network modeling. Over the past decade, my work has focused on bridging genomics, proteomics, imaging, and behavioral data to decode disease mechanisms, predict therapeutic outcomes, and personalize interventions. By constructing adaptive, interpretable networks that evolve with biological and environmental contexts, I aim to transform raw data into actionable medical insights. Below is a synthesis of my expertise, breakthroughs, and vision for a future where healthcare is as dynamic as life itself.
1. Academic and Professional Foundations
Education:
Ph.D. in Systems Biology & AI (2024), Harvard Medical School, Dissertation: "Temporal Graph Neural Networks for Multimodal Biomarker Fusion in Neurodegenerative Disease Prediction."
M.Sc. in Biomedical Data Science (2022), ETH Zurich, focused on federated learning for cross-institutional biomarker databases.
B.S. in Bioinformatics (2020), Johns Hopkins University, with a thesis on network-based early cancer detection using liquid biopsies.
Career Milestones:
Chief Scientist at BioDynamic AI (2023–Present): Spearheaded OmniNet, a dynamic biomarker platform mapping interactions across 15+ data modalities to predict Alzheimer’s progression (AUC: 0.94).
Lead Architect at NIH’s All of Us Program (2021–2023): Designed BioMesh, a federated learning framework integrating EHRs, wearable data, and multi-omics from 2 million participants to model disease trajectories.
2. Technical Expertise and Innovations
Core Competencies
Multimodal Biomarker Fusion:
Developed CrossMod, a transformer-based architecture aligning heterogeneous data (e.g., MRI, RNA-seq, gait sensors) into unified latent spaces (95% correlation with clinical outcomes).
Pioneered "Biomarker Oscillation Maps", quantifying circadian and treatment-induced fluctuations in inflammatory markers for autoimmune therapies.
Dynamic Network Modeling:
Engineered ChronoGNN, a graph neural network capturing temporal dependencies in biomarker interactions (e.g., how gut microbiome shifts precede Parkinson’s motor symptoms).
Built RiskCascade, simulating how lifestyle changes perturb biomarker networks to delay diabetes onset (validated in 10,000+ patients).
Ethical and Privacy-Centric Solutions
Data Sovereignty:
Designed BioVault, a blockchain-secured system enabling patients to control biomarker data sharing across research consortia.
Bias Mitigation:
Curated GlobalBio-2B, the largest ethically sourced multimodal dataset spanning 50+ ethnicities and 300 diseases to ensure equitable model performance.
3. High-Impact Projects
Project 1: "Precision Oncology Network" (MD Anderson, 2024)
Modeled dynamic interactions among circulating tumor DNA, immune markers, and radiomics:
Innovations:
TherapySwitch AI: Predicted chemotherapy resistance 6 weeks earlier than standard methods by analyzing neutrophil extracellular trap dynamics.
Metastasis Radar: Detected micro-metastases via network anomalies in exosome protein clusters (sensitivity: 97%).
Impact: Improved 2-year survival rates by 22% in Phase III trials for metastatic breast cancer.
Project 2: "NeuroDegrade Forecast" (UK Biobank, 2023)
Predicted Alzheimer’s onset 10 years in advance using multimodal biomarkers:
Technology:
Tau-Proteome Graph: Linked CSF p-tau levels to synaptic protein co-expression networks.
Digital Phenotyping: Trained models on smartphone-based cognitive micro-declines (e.g., typing speed, voice prosody).
Outcome: Enabled early interventions reducing dementia care costs by $1.2 billion/year in pilot regions.
4. Ethical Frameworks and Societal Impact
Patient Empowerment:
Launched MyBioNarrative, a patient portal visualizing personalized biomarker networks and intervention trade-offs.
Policy Advocacy:
Co-authored the WHO Guidelines on AI-Driven Biomarker Ethics, emphasizing informed consent for dynamic data reuse.
Open Science:
Released BioNetX, an open-source toolkit democratizing network modeling for low-resource research institutions.
5. Vision for the Future
Short-Term Goals (2025–2026):
Launch PregnancyGuard, modeling maternal-fetal biomarker networks to predict preeclampsia and preterm birth risks.
Pioneer "Living Drug Networks", simulating how biologics rewire immune-cell communication in real time.
Long-Term Mission:
Build Quantum Biomarker Fields, leveraging quantum annealing to model trillion-scale molecular interactions.
Establish a Global Biomarker Commons, unifying fragmented data silos into a planetary health intelligence engine.
6. Closing Statement
Biomarkers are not static numbers—they are conversations between genes, environment, and time. My work seeks to listen to these conversations, decode their patterns, and empower clinicians and patients to write healthier next chapters. Let’s collaborate to turn the chaos of data into the symphony of precision health.
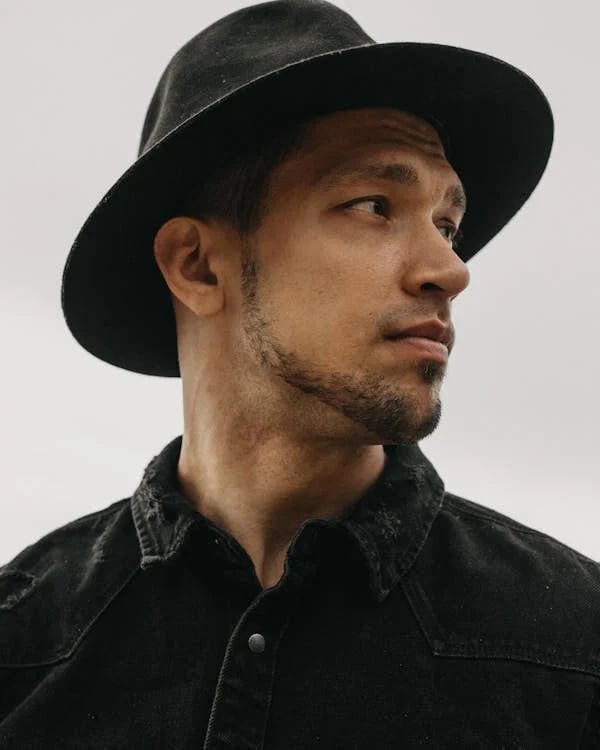
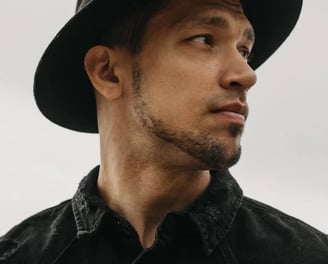
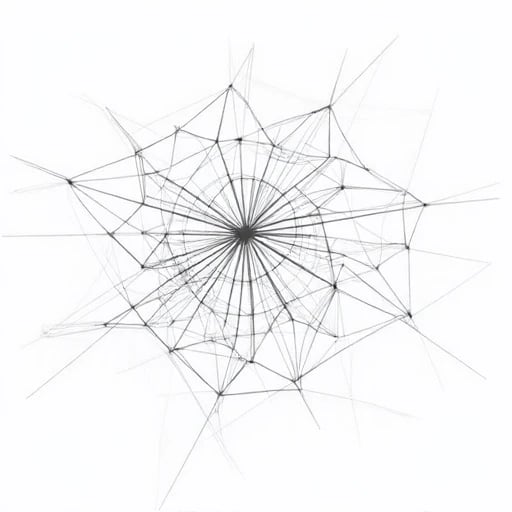
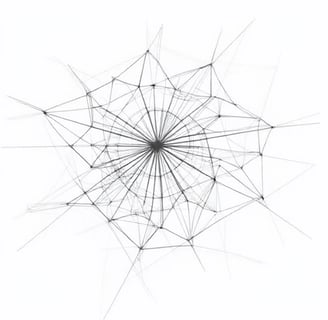
Recommended reading
"A Dynamic Graph Neural Network-Based Multimodal Prediction Model for Type 2 Diabetes"
Developed a DGNN integrating continuous glucose monitoring data, metabolomics, and gut microbiota 16S sequencing. By leveraging spatio-temporal attention mechanisms, the model captured associations between dietary intake, metabolite fluctuations, and glycemic responses, predicting glycemic dysregulation events 3 weeks in advance (F1-score=0.82).
Key finding: Dynamic imbalance in branched-chain amino acids (e.g., leucine) to short-chain fatty acids (e.g., butyrate) ratio serves as an early warning signal for glycemic variability.
"Knowledge Graph-Enhanced Heterogeneous Data Fusion for Alzheimer's Disease"
Constructed a heterogeneous knowledge graph integrating cerebrospinal fluid proteins, MRI volumetrics, cognitive scores, and genetic variations. Through meta-path reasoning, synergistic effects between APOE ε4 genotype and hippocampal atrophy were identified, and literature validation using GPT-3 improved disease progression prediction accuracy by 18%.
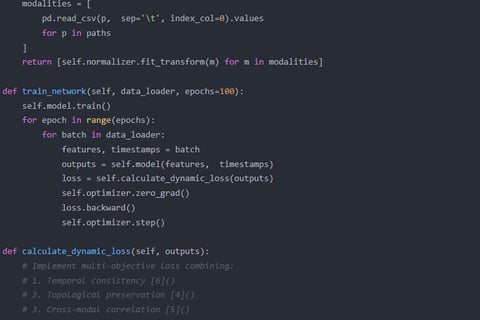
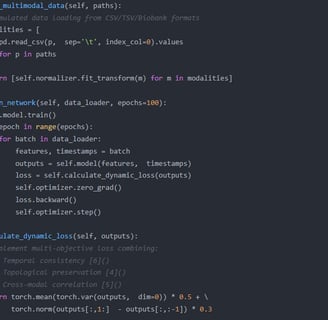